Tembo’s Blog
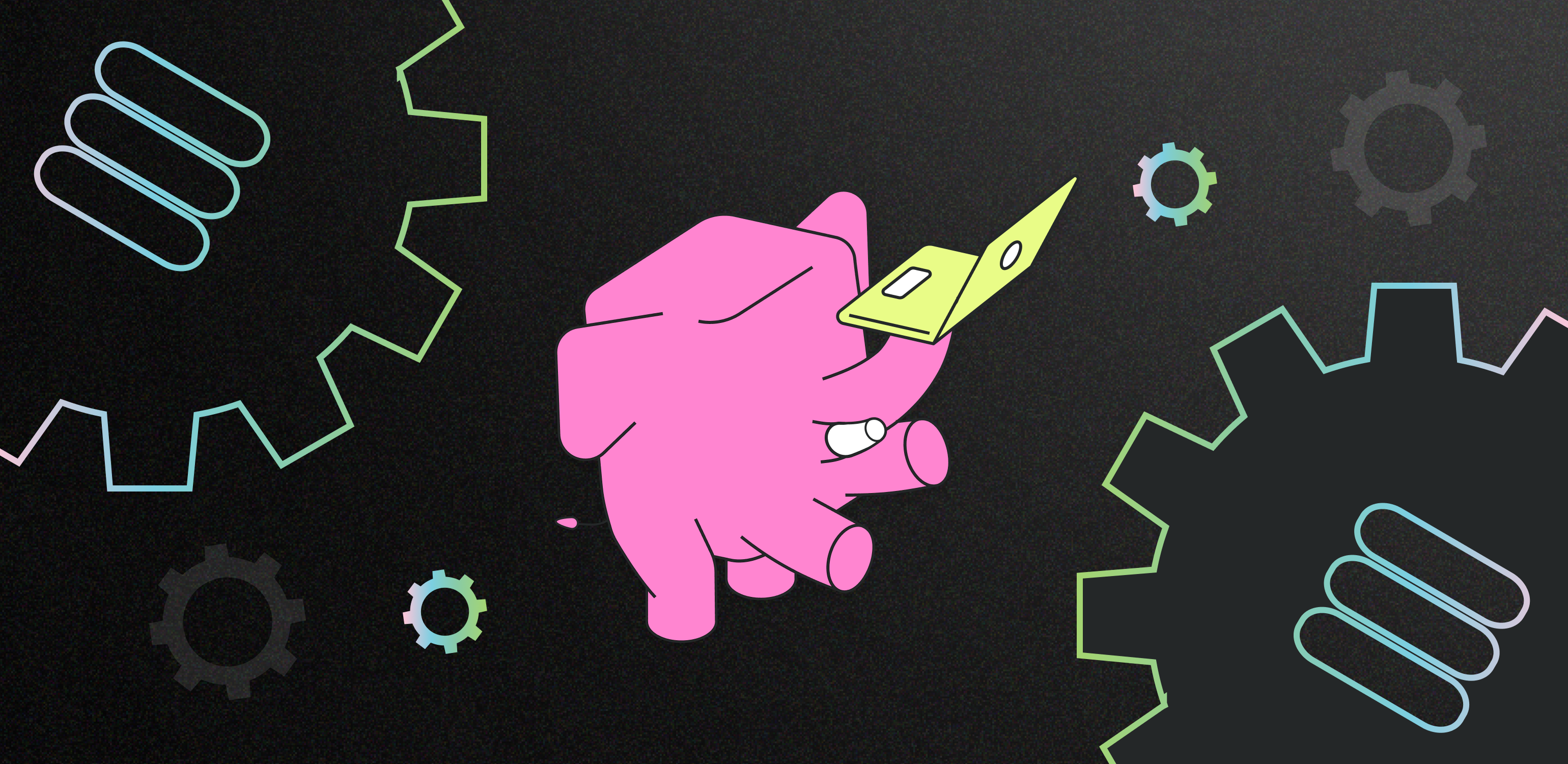
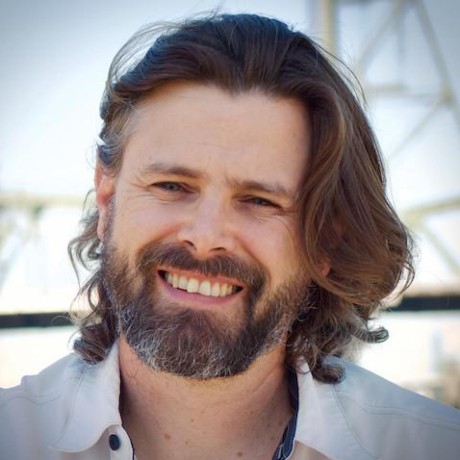
David E. Wheeler
Principal Architect
What’s New on the PGXN v2 Project
6 min read
Sep 11, 2024
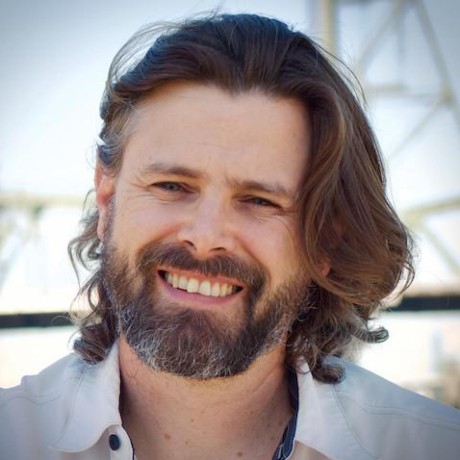
David E. Wheeler
Principal Architect
What’s Happening on the PGXN v2 Project
4 min read
Apr 3, 2024
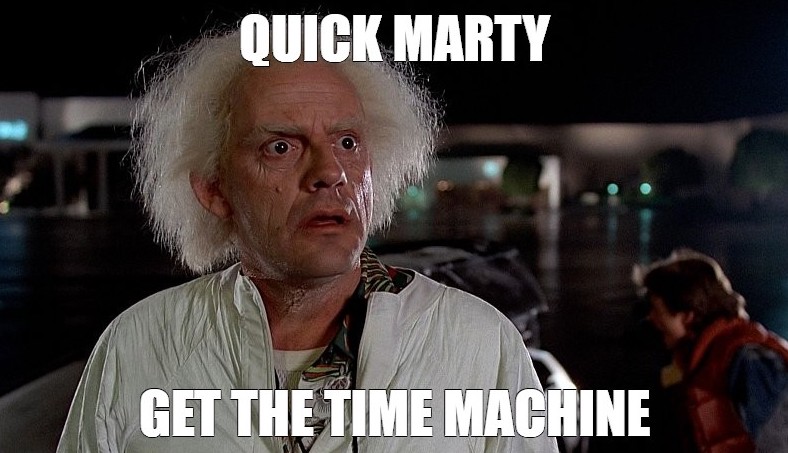
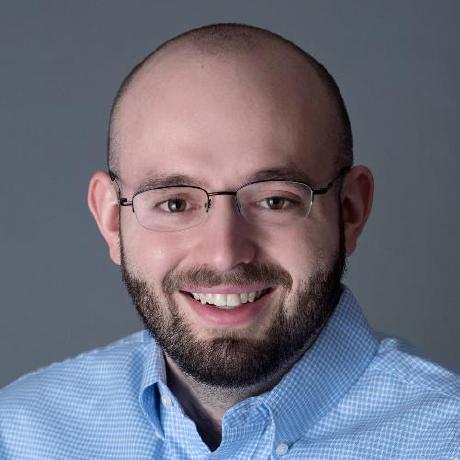
Steven Miller
Founding Engineer
Version History and Lifecycle Policies for Postgres Tables
17 min read
Sep 29, 2023
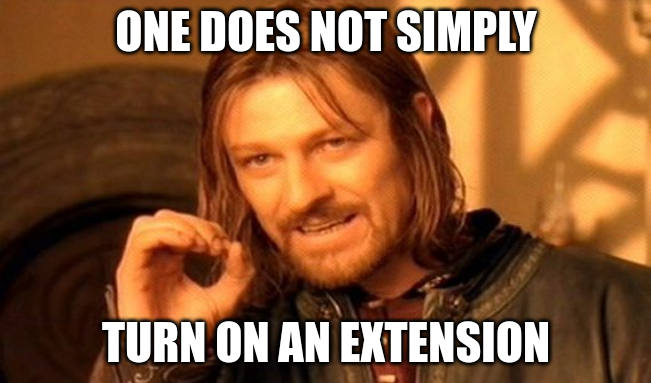
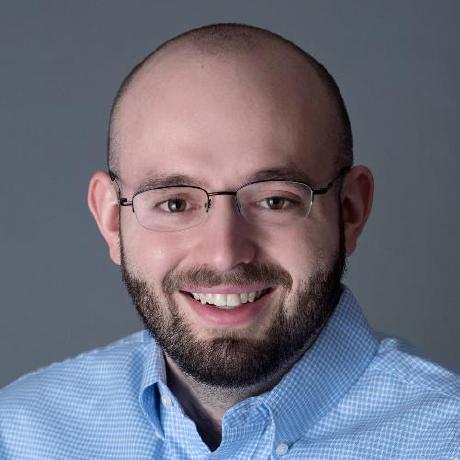
Steven Miller
Founding Engineer
Enter the matrix: the four types of Postgres extensions
12 min read
Sep 14, 2023