Tembo's Blog
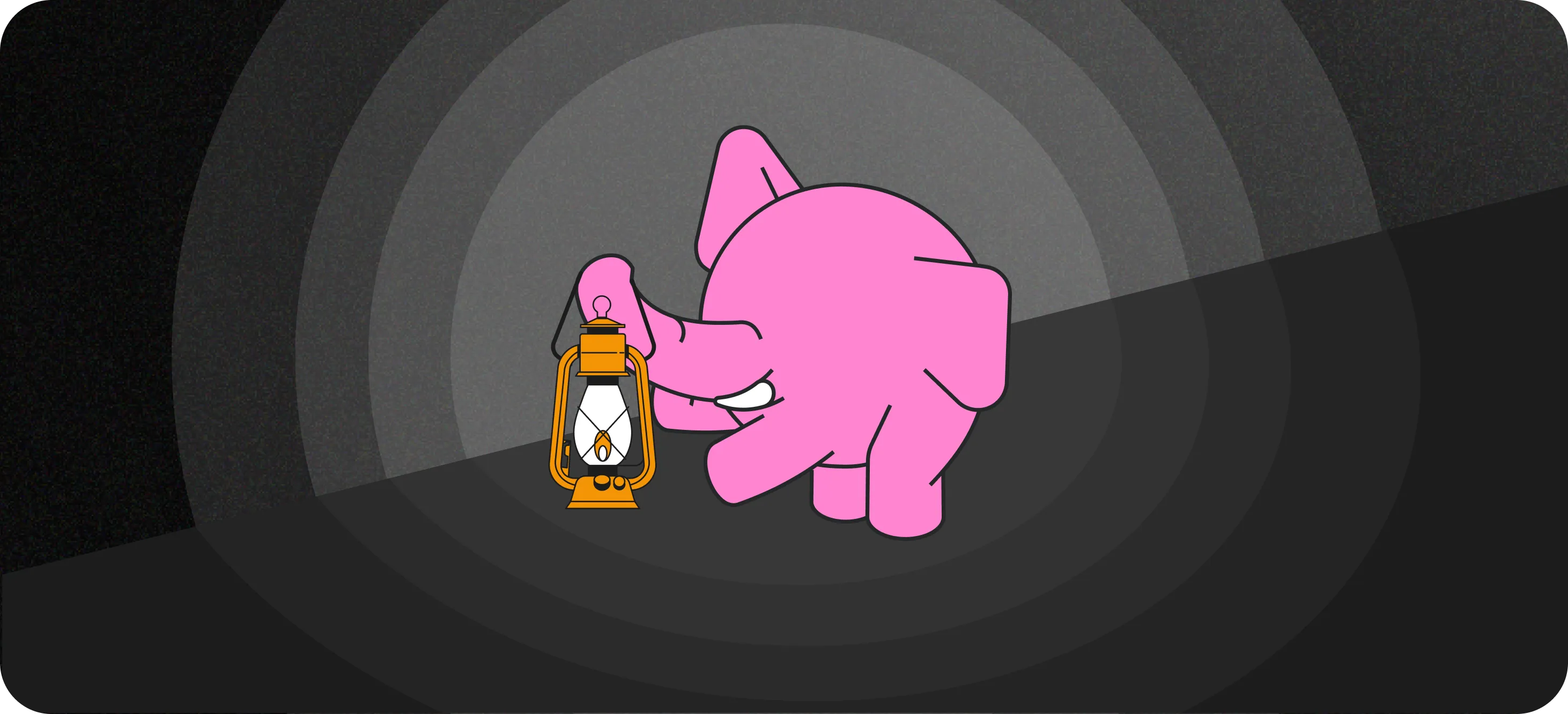
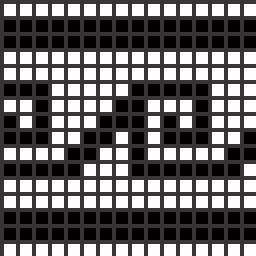
Binidxaba
Community contributor
Pgvector vs Lantern part 2 - The one with parallel indexes
3 min read
Feb 5, 2024
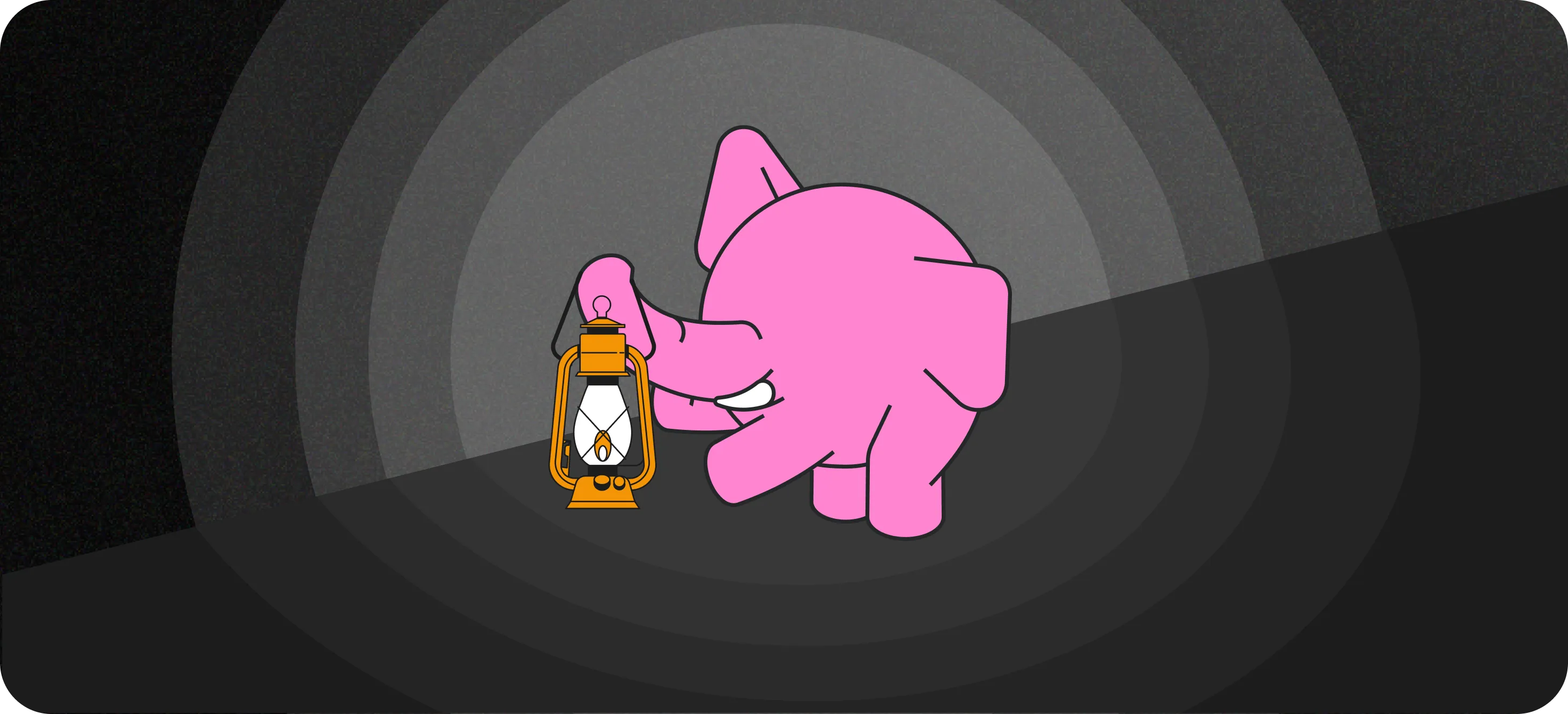
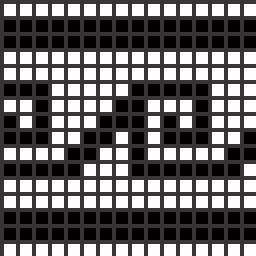
Binidxaba
Community contributor
Benchmarking Postgres Vector Search approaches: Pgvector vs Lantern
6 min read
Jan 17, 2024
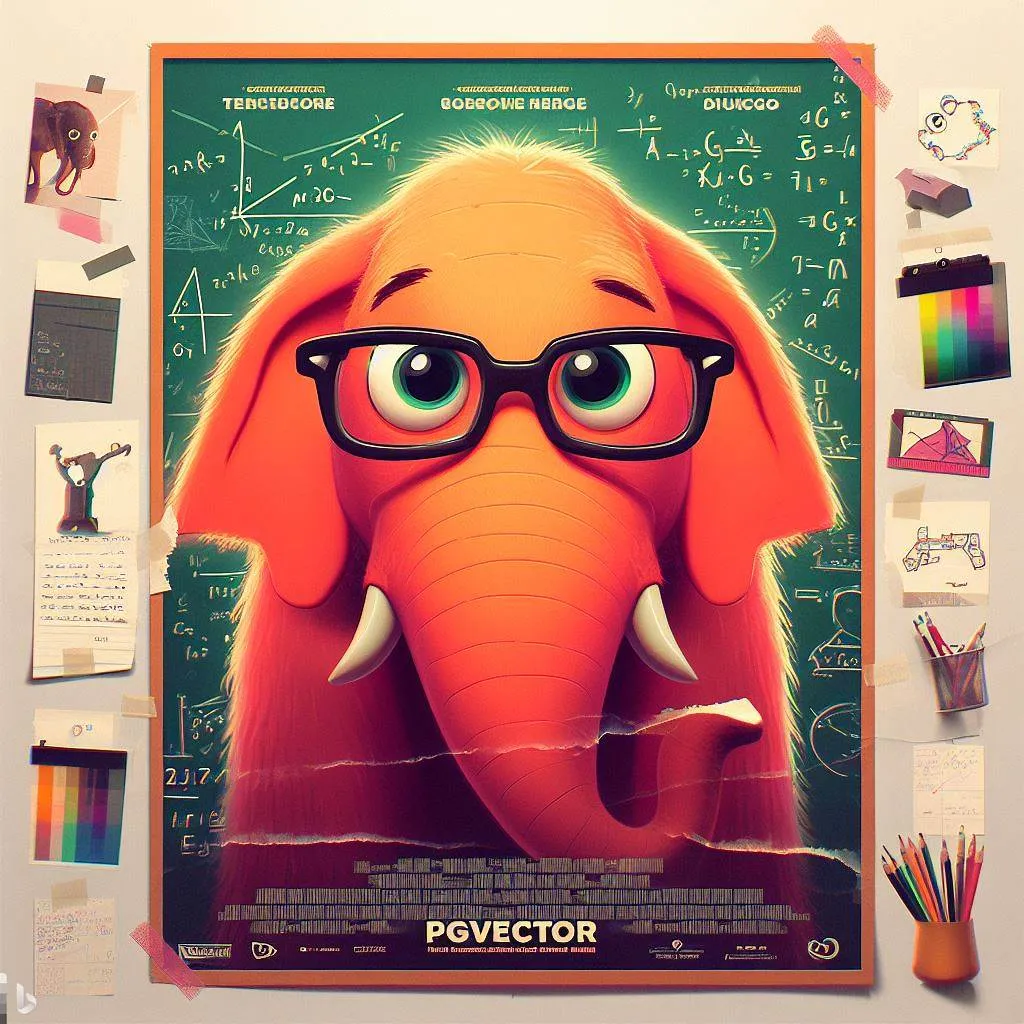
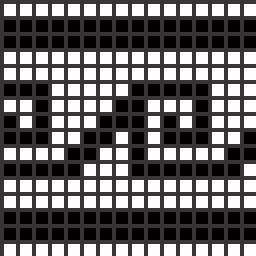
Binidxaba
Community contributor
Vector Indexes in Postgres using pgvector: IVFFlat vs HNSW
10 min read
Nov 14, 2023
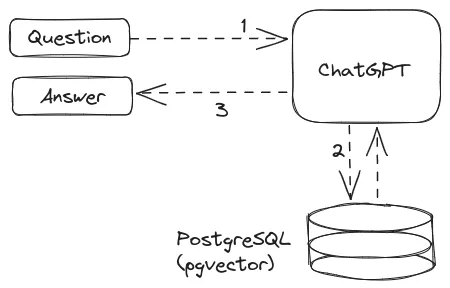
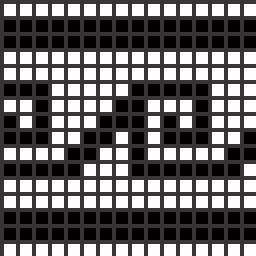
Binidxaba
Community contributor
Unleashing the power of vector embeddings with PostgreSQL
8 min read
Oct 18, 2023